AI sentencing cut jail time for low-risk offenders, but study finds racial bias persisted
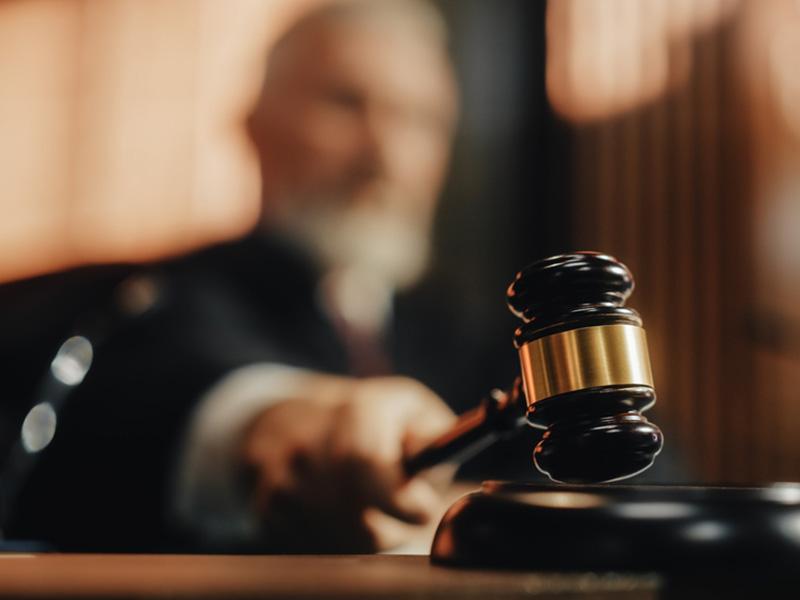
Judges relying on artificial intelligence tools to determine criminal sentences handed down markedly less jail time in tens of thousands of cases, but also appeared to discriminate against Black offenders despite the algorithms' promised objectivity, according to a new study by a researcher at Tulane University's A. B. Freeman School of Business.
Researchers examined over 50,000 drug, fraud and larceny convictions in Virginia where judges used AI software to score each offender's risk of reoffending. The technology recommended alternative punishments like probation for those deemed low risk.
The AI recommendations significantly increased the likelihood low-risk offenders would avoid incarceration — by 16% for drug crimes, 11% for fraud and 6% for larceny. This could help ease the burden on states facing high incarceration costs. Defendants with AI-backed recommendations saw their jail terms shortened by an average of nearly a month — and following AI’s sentencing advice made it more likely offenders wouldn’t land back in jail for repeat offenses.
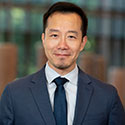
“Recidivism was about 14% when both the AI tool and judges recommended alternative punishments but much higher — 25.71% to be specific — when the AI tool recommended an alternative punishment, but the judge instead opted to incarcerate,” said lead study author Yi-Jen "Ian" Ho, associate professor of management science at the Freeman School. “We can conclude from that that AI helps identify low-risk offenders who can receive alternative punishments without threatening public safety. Overall, it appears clear that following AI recommendations reduces both incarceration and recidivism.”
But Ho also uncovered evidence the AI tools, intended to make sentencing more impartial, may be having unintended consequences.
Judges have long been known to sentence female defendants more leniently than men. Ho found the AI recommendations helped reduce this gender bias, leading to more equal treatment of male and female offenders.
However, when it came to race, judges appeared to misapply the AI guidance. Ho found judges generally sentenced Black and White defendants equally harshly based on their risk scores alone. But when the AI recommended probation for low-risk offenders, judges disproportionately declined to offer alternatives to incarceration for Black defendants.
As a result, similar Black offenders ended up with significantly fewer alternative punishments and longer average jail terms than their White counterparts — missing out on probation by 6% and receiving jail terms averaging a month longer.
“To prevent this, we think better AI system training and feedback loops between judges and policymakers could help. Also, we believe judges should be encouraged to pause and reconsider their sentences when they deviate from AI advice,” Ho said. “They should be aware of unconscious biases that skew discretionary decisions.”
The findings demonstrate AI tools can make sentencing more objective but also perpetuate discrimination if allowed. Ho said it highlights the need for an informed public discussion about AI's risks and benefits before implementing such systems more widely.
“Overall, while AI does appear to bolster certain aspects of justice administration, there continue to be instances where human biases intercede and contradict data-driven risk analysis,” Ho said. “As a result, we think that ongoing legal and ethical oversight regarding AI is essential.”
— Keith Brannon kbrannon@tulane.edu